Past Events
Event Status
Scheduled
Dec. 4, 2015, All Day
Abstract: A fundamental problem to all data-parallel applications is data locality. There are multiple levels of data locality, as a data block can be accessed in local memory or disk, within a rack or a data center, or across data centers. Scheduling with data locality is an affinity scheduling problem with an explosive number of task types and unknown task arrival rates. As a result, existing algorithms do not apply and the recently proposed JSQ-MaxWeight (Wang et. al. 2014) algorithm for two-level locality is delay-optimal only for a special heavy traffic scenario.
Event Status
Scheduled
Nov. 20, 2015, All Day
Abstract: The fast Johnson-Lindenstrauss transform has triggered a large amount of research into fast randomized transforms that reduce data dimensionality while approximately preserving geometry. We discuss uses of these fast transforms in three situations. In the first, we use the transform to precondition a data matrix before subsampling, and show how for huge data sets, this leads to great acceleration in algorithms such as PCA and K-means clustering. The second situation reconsiders the common problem of sketching for regression.
Event Status
Scheduled
Nov. 18, 2015, All Day
Abstract: The Intensive Care Unit (ICU) is playing an expanding role in acute hospital care, but the value of many treatments and interventions in the ICU is unproven, and high-quality data supporting or discouraging specific practices are sparse. Much prior work in clinical modeling has focused on building discriminating models to detect specific coded outcomes (e.g., hospital mortality) under specific settings, or understanding the predictive value of various types of clinical information without taking interventions into account.
Event Status
Scheduled
Nov. 16, 2015, All Day
Abstract: Ubiquitous sensors generate prohibitively large data sets. Large volumes of such data are nowadays generated by a variety of applications such as imaging platforms and mobile devices, surveillance cameras, social networks, power networks, to list a few. In this era of data deluge, it is of paramount importance to gather only the data that is informative for a specific task in order to limit the required sensing cost, as well as the related costs of storing, processing, or communicating the data.
Event Status
Scheduled
Nov. 13, 2015, All Day
Abstract: Submodular functions capture a wide spectrum of discrete problems in machine learning, signal processing and computer vision. They are characterized by intuitive notions of diminishing returns and economies of scale, and often lead to practical algorithms with theoretical guarantees.
In the first part of this talk, I will give a general introduction to the concept of submodular functions, their optimization and example applications in machine learning.
Event Status
Scheduled
Nov. 6, 2015, All Day
Abstract: Many machine learning tasks can be posed as structured prediction, where the goal is to predict a labeling or structured object. For example, the input may be an image or a sentence, and the output is a labeling such as an assignment of each pixel in the image to foreground or background, or the parse tree for the sentence.
Event Status
Scheduled
Oct. 30, 2015, All Day
no results
Event Status
Scheduled
Oct. 23, 2015, All Day
Abstract: Sampling is a standard approach to big graph analytics. Buta good sample need to represent graph properties of interest with aknown degree of accuracy. This talk describes a generic tunablesampling framework, graph sample and hold, that applies to graphstream sampling in which edges are presented one at a time, and fromwhich unbiased estimators of graph properties can be produced inpost-processing. The talk also describes the performance of the methodon various types of graph, including social graphs, amongst others.
Watch the full presentation on the WNCG YouTube Channel.
Event Status
Scheduled
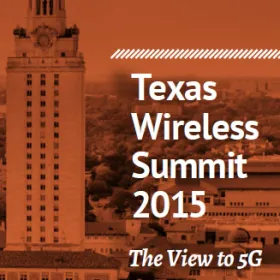
Oct. 16, 2015, All Day
The 13th annual Texas Wireless Summit (TWS) provides a forum on emerging technology and business models for industry leaders and academics. Hosted by the University of Texas at Austin's Wireless Networking and Communications Group (WNCG), the Summit offers direct access to cutting-edge research and innovations from industry leaders, investors, academics and startups.
Event Status
Scheduled
Oct. 7, 2015, All Day
Abstract: Given samples from an unknown distribution, p, is it possible to distinguish whether p belongs to some class of distributions C versus p being far from every distribution in C, by at least ε in total variation distance? This fundamental question has received substantial attention in Statistics and Computer Science. Nevertheless, even for basic classes of distributions such as monotone, log-concave, unimodal, or product, the optimal sample complexity is unknown. We provide optimal testers for these families.
(joint work with Jayadev Acharya and Gautam Kamath).